đĄ Artificial intelligence â where are we at?
đĄ Artificial intelligence â where are we at?
Paris, 22 January, 8.30am: the panel at this early bird meeting, organised by French power supplier ENGIE, discussed artificial intelligence (AI) from all angles. They debunked a few myths too!
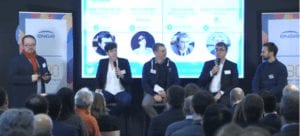
Right now, AI is on the crest of a wave. Yet dreams of intelligent machines date back centuries. In the Middle Ages there was Albert the Great and his famous âtalking headâ, which was reputed to correctly answer any question put to it. In the 18th century, a particularly popular period for automatons (or robots), Johann Maelzel created an illusion with the Turk, a chess playing automaton. Then in 1818, the publication of Mary Shelleyâs novel Frankenstein raised questions, often uncomfortable, such as âwhat does it mean to be human?â âWhat is the nature of intelligence?â âNatural progression or threat?â
Fast forward to the 21st century and we have the chatbots Alexa and Siri, as well as Sophia, the humanoid robot.
From Frankenstein to Sophia. âThe idea behind AI is to create oneself what God created. Becoming God and creator is a dream that has been around for a very long time,â says Erik Orsenna, economist and author.
Machine learning and data
According to French philosopher and AI researcher Alban Leveau-Vallier, todayâs buzz represents the âthird wave of AIâ since the 1950s, the decade when talk and research into the technology really began in earnest. Given the previous two âwavesâ crashed after researchers failed to deliver on their many promises, whatâs different about this one? âMainly machine learning,â says Mr Leveau-Vallier, âwhich, since 2010-2012, has triggered a new influx of research, innovation, and funding. The advantage of machine learning is that you can show the computer a vast amount of data and it does the task required of it. This makes starting out and testing applications very easy.â
For Alexia Audevart, data & enthusiasm, founder of Datactik, the two main approaches to AI to date are the âsymbolicâ and the âconnectionistâ.
âThe âsymbolicâ approach in the 1980s was based on trying to model the expertise of an expert in order to make deductions,â she explains. âBut it involved modelling all the facts and all the rules, so proved hugely complicated.â
In contrast, the âconnectionistâ method, based on machine learning, is more straightforward. âThe machine learns without being programmed; in other words, the machine is given a load of data and gets on with the task. It is small branch of deep learning, bio-inspired by the human brain.â
Despite the current excitement over AI, plenty of questions are still up in the air. Especially when it comes to data. Like how to build a âgoodâ database, and what exactly does this mean? âWhat is an ethical database?â queries Mr Leveau-Vallier. âDoes it mean no discrimination of the data to give a fair representation of society? But then, who decides how to correct the database so the algorithms recalibrate themselves to create this âgood/rightâ database?â
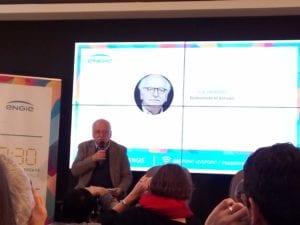
AI in action
ENGIE has had its finger on the AI pulse for many years, according to the groupâs digital innovation officer Etienne Gehain. âWe’ve been using it for applications like predictive maintenance or to anticipate any operational problems. For instance, through image analysis itâs possible to identify âhot spotsâ on photovoltaic panels and take action before any disfunctions occur. Another use case is customer relations, with the chatbots Alexa and Google Assistant.â
Today, the company is drawing on deep learning â machine learning enhanced by Neural Networks, algorithms that mimic the biological structure of the brain â to further develop its AI IT tools. âWe view deep learning as a new way of computing for ENGIE,â says Mr Gehain. “Itâs about learning, rather than programming, to create value from data and has massively extended the field of AI.
“At the same time, we are aware of the importance of adopting a more conscious approach to data. In other words, working to quantify the data, to label and put it in context, as well as exploring how to share it to create value.â
The first AI initiative in France, the France IA plan, was launched in January 2017 by president Francois Hollande. It was taken up later that year by Cédric Villani, mathematician and member of the French Parliament. In March 2018, Mr Villani delivered For a meaningful Artificial Intelligence, a 154-page roadmap for the French AI strategy.
France, the AI place to be?
On the topic of AI research in France, it seems the combination of French talent and salaries lower than in the US appeal to the GAFA tech giants: both Facebook and Google have opened research labs in Paris. Similarly, the French touch is attracting major industry groups.
In April 2018, Siemens announced its partnership with the French Alternative Energies and Atomic Energy Commission (CEA). Together they plan to open a collaborative workspace for research into data analysis and AI. Baptised The MindSphere Center, it will be located on the Paris-Saclay innovation campus.
At the same time, brain drain is already a reality, with French researchers being lured abroad by lucrative pay packages.
âSalaries are one thing, but another is promising employees they will be able to continue learning and stay at the top of their game,â points out Mr Gehain. âItâs important we can give young researchers these guarantees.â
>> Back to the future <<<
Will AI become the new way of doing computing, the new normal? âWell it depends on how our imagination evolves,â reckons Mr Leveau-Vallier. âWe expected technology would bring order to the world, but instead we are seeing the opposite effect since it is reconfiguring the world and surprising us.â
Will this reconfiguration include robots replacing humans in the workplace, a fear much channelled in the media? Mr Orsenna seeks to reassure: âItâs all down to the irreducible in life. Medical diagnostics performed using big data are more accurate than when done by doctors. So, will doctors be out of a job in the future? No, because they will do the tasks machines canât.â
To make sense of the reality of AI, he suggests looking at the semantics. âIs artificial intelligence the right term we should be using? Why this official âappellation dâorigine contrĂŽlĂ©eâ labelling? Also, whatâs âdeepâ about deep learning? Wouldnât âauto-learningâ be more accurate? Questioning the terminology in this way can help clarify our thinking about AI.â
âWhy does everyone struggle to clearly define AI? Because we have yet to define human intelligence,â adds Mr Leveau-Vallier. âRight now, AI really describes deep learning. Maybe in two decades the term will refer to another variant.â
Given the past failures of AI to live up to expectations, he is cautious about predicting the outcomes of this 3rd wave. âFor the past 70 years, we have been promising AI will come âin 20 yearsâ. Itâs like in âThrough the Looking Glassâ [book by Lewis Carroll, published in 1871] â âjam yesterday, tomorrow, but not todayâ.â
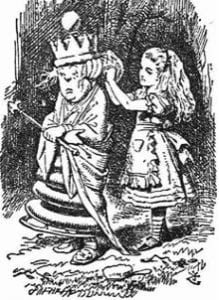
Mr Leveau-Vallier believes the current AI trend will still âdeliver no jam tomorrow, nor in five, nor in 10 years,â yet acknowledges it is stirring up the field, with interesting developments and innovative advances, like facial recognition.
âTomorrow is radically unpredictable,â he sums up, using the flying cars and phone boxes in the 1982 sci-fi film Blade Runner to illustrate his point. âIn 2019, the only thing that has happened is that phone boxes have disappeared due to the dominance of the smartphone, but we donât have flying cars or androids, yet!â
Watch the video (in French)
Cover photo and screenshots of speakers: ENGIE